Understanding Predictive Content Analytics: Key Insights and Benefits
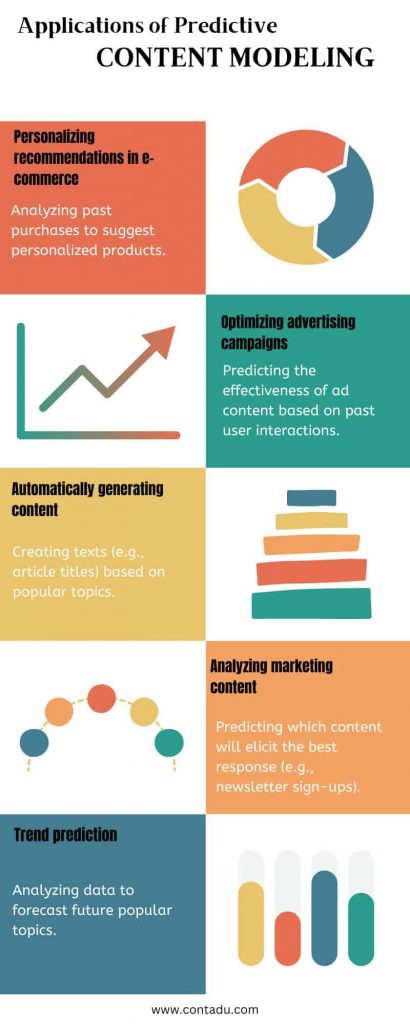
Today where gigantic amounts of textual data are generated every day, predictive content modeling is gaining importance as one of the key analytical tools. Applications of this technology go far beyond standard data analysis, offering advanced predictions based on available content. What exactly is predictive content modeling and how can it be used in business and research?
What is Predictive Content Modeling?
Predictive content modeling is an analytical technique that allows for the forecasting of future trends, user behaviors, and other variables based on the analysis of existing textual data. This can include news articles, social media posts, emails, customer comments, conversation transcripts, and other forms of written information.
The process involves using artificial intelligence (AI) and machine learning (ML) methods to identify patterns in texts that may indicate future changes. Natural Language Processing (NLP) algorithms play a crucial role here, enabling computers to “understand” and analyze content in a manner similar to humans.
How Does Predictive Content Modeling Work?
Predictive content modeling is the process of analyzing textual data to forecast future events based on discovered patterns. Initially, relevant data is collected, such as social media posts, reviews, or reports. Then, using Natural Language Processing (NLP), algorithms analyze this data, identifying key topics and meanings. The next step involves applying machine learning to create models that predict future events, such as changes in customer preferences or market trends.
What Are the Benefits of Predictive Analysis?
Predictive data analysis allows for forecasting future events based on historical data and advanced algorithms, offering numerous benefits:
- Making more informed decisions.
- Precisely tailoring offers to customer needs.
- Optimizing processes and saving costs.
- Early detection of threats.
- Increasing the effectiveness of sales and marketing campaigns.
- Preventing customer churn.
- Anticipating trends and better adapting to market changes.
- Improving efficiency, profitability, and customer relationships.
Applications of Predictive Content Modeling
Predictive content modeling is used in various fields, from marketing to market research, and in sectors such as finance, media, and technology. Here are some examples of its applications:
How to Start Using Predictive Analytics?
Starting with predictive analytics might seem complex, but it can be simplified. Here’s how to do it step by step:
- Define Your Goals – First, consider what you want to predict, e.g., how much you will sell next month, how many customers will churn, or where you can save money.
- Collect Data – Your data is the foundation. This can be anything: sales reports, customer information, CRM records. Ensure that the data is clean and organized (without errors or missing values).
- Choose Tools – You can use various tools for analysis, such as Python, R, or IBM Watson. Choose something that fits your team and capabilities.
- Build the Model – Now, create a model that will predict future events. It’s like a machine that learns from past data to forecast what will happen in the future.
- Test the Model – Test your model on data that was not used in its construction to ensure it performs well and does not just predict what it already knows.
- Implement – Once the model works correctly, put it into operation. Monitor its predictions to ensure they are accurate and update it as needed.
- Analyze Results – Use the analysis results to make better business decisions. Present results clearly and understandably, e.g., in reports or charts.
- Improve the Model – The world changes, so should your model. Update data, test new approaches, and check how to predict the future even better.
This step-by-step approach will help you start using predictive analytics in a clear and effective way.
What Tools Are Available for Implementing Predictive Analytics?
Starting with predictive analytics involves choosing the right tools and technologies aligned with the company’s goals. Many software solutions cater to various predictive analysis needs. Leading platforms like IBM SPSS, SAS, and Tableau offer advanced data visualization and predictive modeling features, as well as support for data exploration and machine learning. Cloud solutions such as Google Cloud AI and Microsoft Azure provide scalable options for companies that want to implement predictive analytics without substantial initial investments.
How Contadu Helps with Predictive Analytics.
Contadu is a tool that helps companies create better content by leveraging predictive analytics. But what does this mean for the average user? It uses artificial intelligence (AI) to predict which content will be most effective in the future.
Predictive analytics in Contadu involves analyzing data on current trends and topics of interest to users. This enables the tool to predict which content might be popular in a week, a month, or even a year. This means companies can plan and create content in advance, before it becomes widely sought after.
Contadu also assists with competitive analysis – it suggests which topics are already well-covered by other companies and where to find so-called “content gaps.” This helps in creating unique and valuable articles that better meet readers’ needs.
With automatic optimization, Contadu analyzes whether the content is well-matched to SEO requirements (i.e., if it will be easily found on Google). Predictive analysis in this context helps in adapting content to changing trends and user needs. All of this happens in real-time – the tool suggests changes that can be made to make content more effective.
In this way, Contadu supports companies in creating better content faster and more efficiently.